ShapeTalk:
A Language Dataset
and Framework for 3D Shape Edits and Deformations
Abstract
Editing 3D geometry is a challenging task requiring specialized skills. In this work, we aim to facilitate the task of editing the geometry of 3D models through the use of natural language. For example, we may want to modify a 3D chair model to “make its legs thinner” or to “open a hole in its back”. To tackle this problem in a manner that promotes open-ended language use and enables fine-grained shape edits, we introduce the most extensive existing corpus of natural language utterances describing shape differences: ShapeTalk. ShapeTalk contains over half a million discriminative utterances produced by con- trasting the shapes of common 3D objects for a variety of object classes and degrees of similarity. We also introduce a generic framework, ChangeIt3D, which builds on ShapeTalk and can use an arbitrary 3D generative model of shapes to produce edits that align the output better with the edit or deformation description. Finally, we introduce metrics for the quantitative evaluation of language-assisted shape editing methods that reflect key desiderata within this editing setup. We note, that our modules are trained and deployed directly in a latent space of 3D shapes, bypassing the ambiguities of “lifting” 2D to 3D when using extant foundation models and thus opening a new avenue for 3D object-centric manipulation through language.
The ShapeTalk Dataset
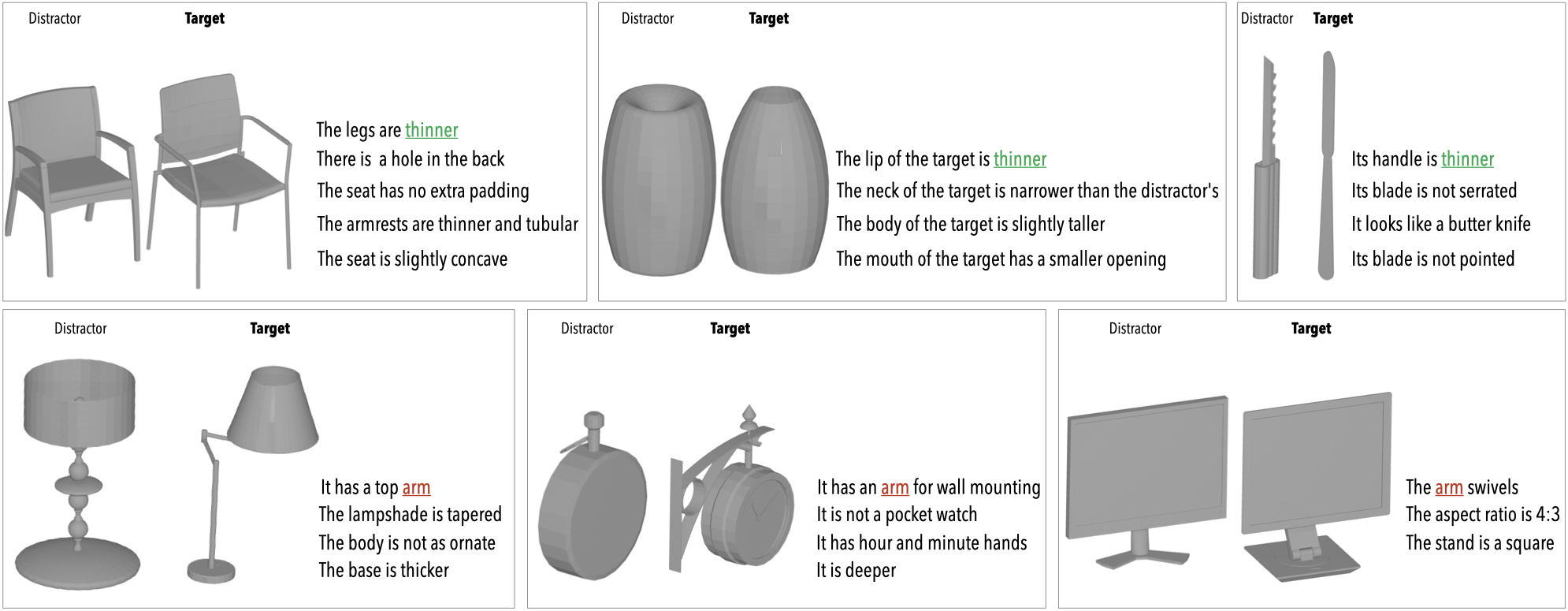
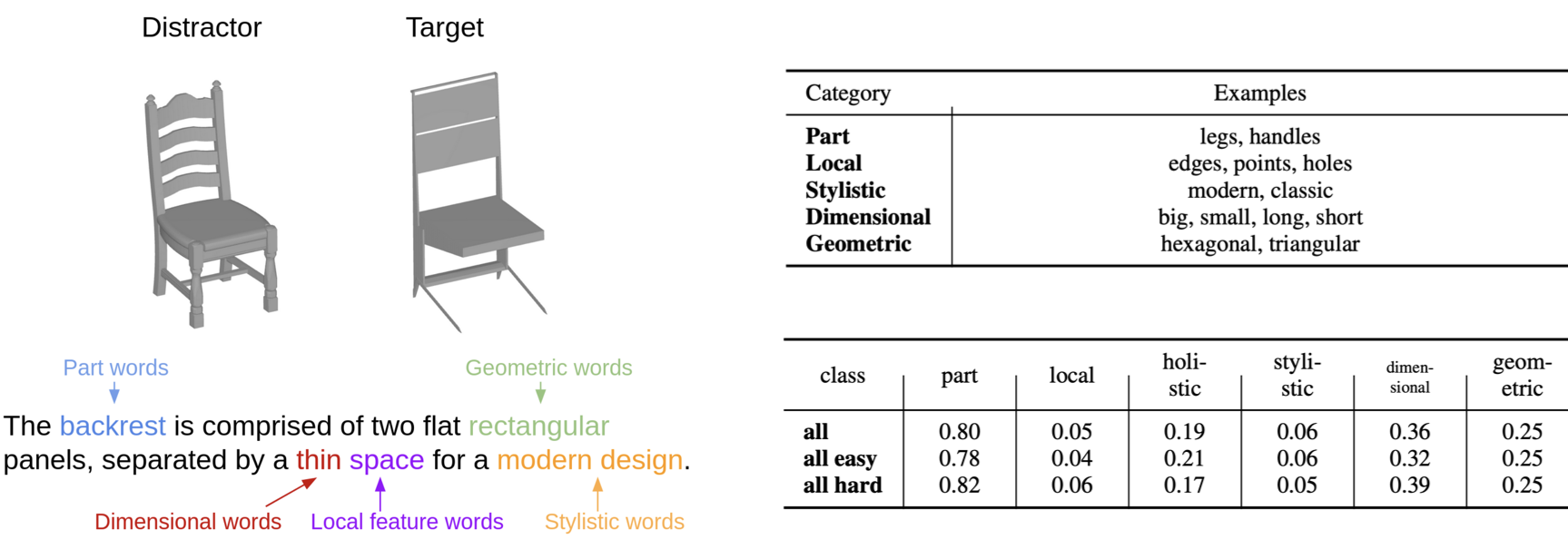
Browse
You can browse the ShapeTalk annotations here.
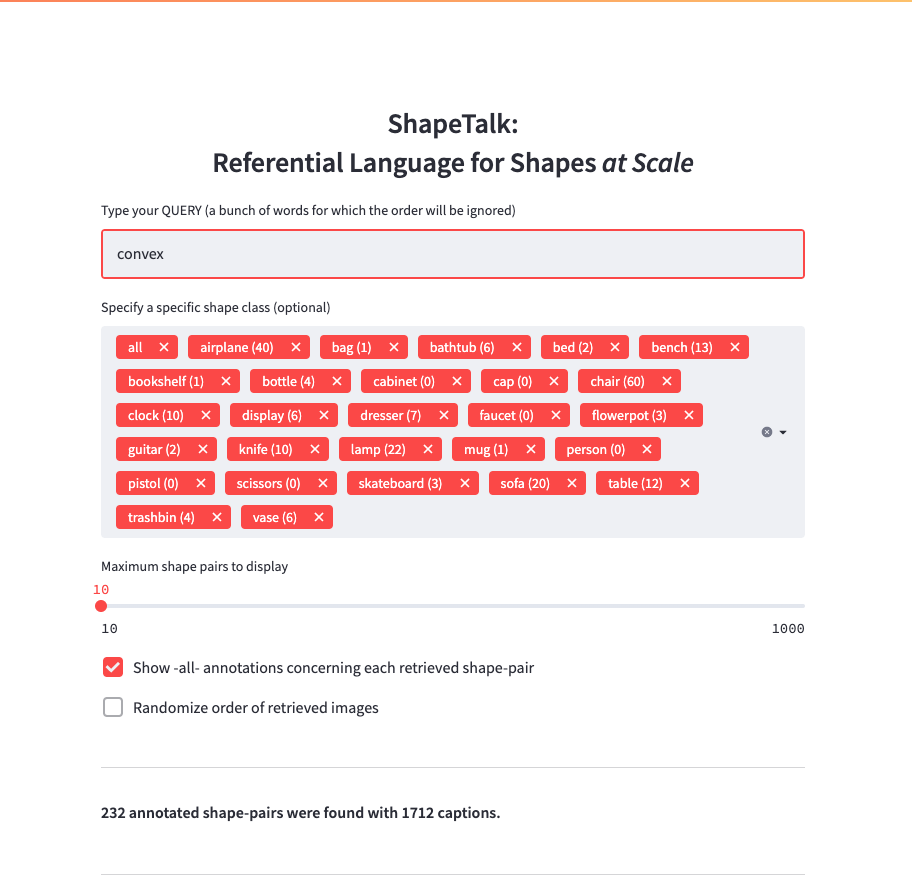
License & Download
- The ShapeTalk dataset is released under the ShapeTalk Terms of Use.
- To download the ShapeTalk dataset please first fill out this form, accepting the Terms of Use.
ChangeIt3D Architecture
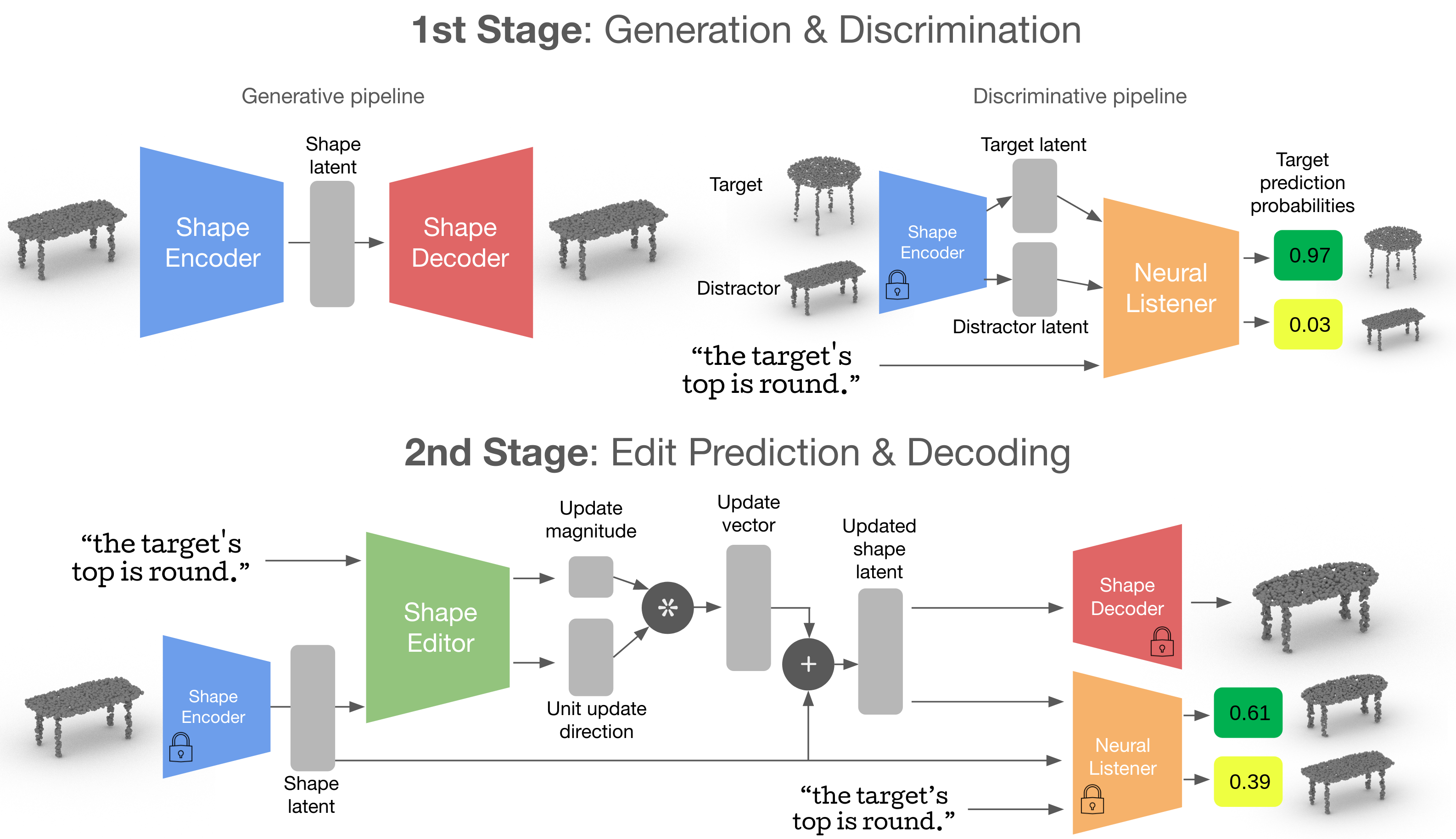
Qualitative Results
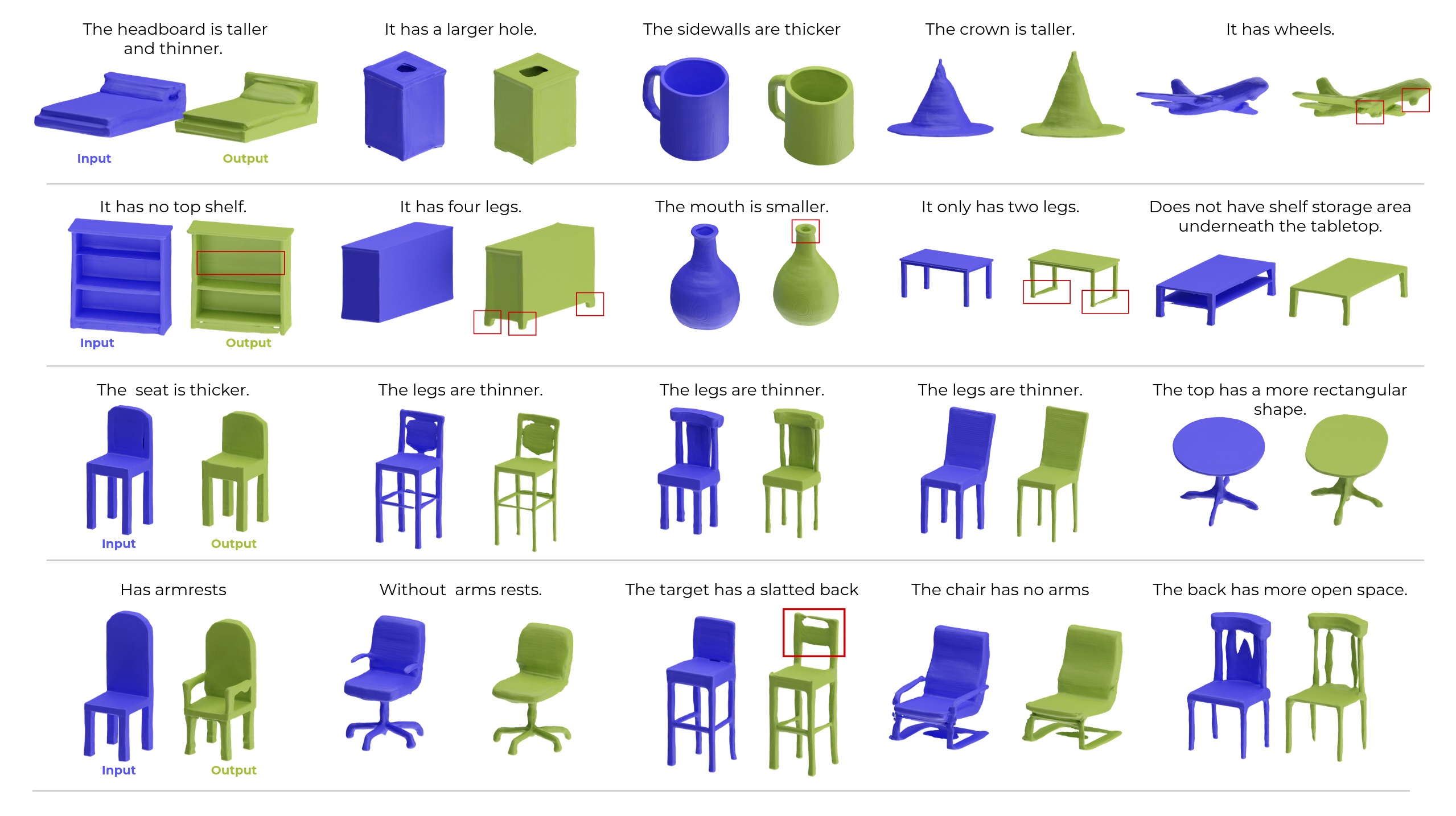
Citations
If you find our work useful in your research, please consider citing:
@inproceedings{achlioptas2023shapetalk, title={{ShapeTalk}: A Language Dataset and Framework for 3D Shape Edits and Deformations}, author={Achlioptas, Panos and Huang, Ian and Sung, Minhyuk and Tulyakov, Sergey and Guibas, Leonidas}, booktitle={Conference on Computer Vision and Pattern Recognition (CVPR)}, year={2023}}
Also, if you you use the ShapeTalk dataset please also consider citing our previous paper/data: ShapeGlot, which was critical in building and analyzing ShapeTalk:
@inproceedings{achlioptas2019shapeglot, title={{ShapeGlot}: Learning Language for Shape Differentiation}, author={Achlioptas, Panos and Fan, Judy and Hawkins, Robert and Goodman, Noah and Guibas, Leonidas}, booktitle = {International Conference on Computer Vision (ICCV)}, year={2019}}
Acknowledgements
This work is funded by a Vannevar Bush Faculty Fellowship, an ARL grant W911NF-21-2-0104, and a gift from Snap corporation. Panos Achlioptas wish to thank for their advices and help the following researchers: Iro Armeni (data collection), Nikos Gkanatsios (neural-listening), Ahmed Abdelreheem (rendering), Yan Zheng and Ruojin Cai (SGF deployment), Antonia Saravanou and Mingyi Lu (relevant discussions) and Menglei Chai (CLIP-NeRF). Last but not least, the authors want to emphasize their gratitude to all the hard-working Amazon Mechanical Turkers without whom this work would be impossible.